In recent years, the development of technologies such as the Internet, the Internet of Things, and Wearable Sensor Networks (WSN) has provided a new approach for the long-distance rehabilitation training and evaluation of patients with stroke, but how To ensure long-term stable operation of the equipment has yet to be resolved. 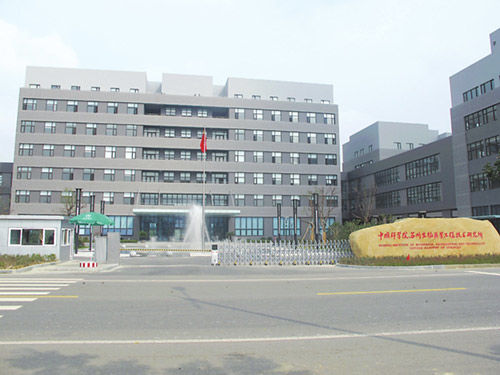
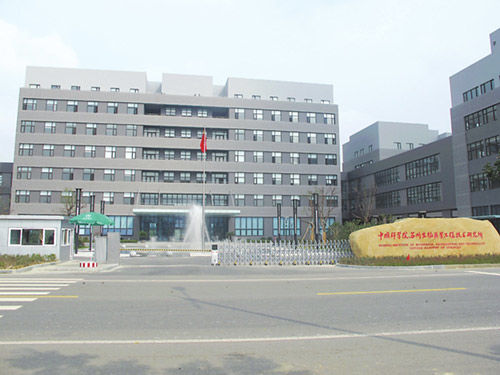
Compressed Sensing (CS) is a new theoretical framework for achieving the purpose of compression while sampling. It can sample the signal at a lower frequency and reconstruct the signal with high probability. This provides a possible solution to long-running low power consumption of wearable devices.
The Guo Liquan Research Group of the Institute of Photonics Research, Suzhou Institute of Biomedical Engineering and Technology, Chinese Academy of Sciences, developed a wearable sensor device based on various MEMS sensors (acceleration, gyroscopes, magnetometers, bending sensors, etc.) and built a ZigBee wireless protocol. Wireless sensor network platform.
On this basis, a long-distance rehabilitation training and assessment system was developed using internet technology, which can be used for the training and assessment of home-based or community-based rehabilitation for stroke patients. In order to reduce the amount of wireless data transmitted by wearable sensor devices and extend their endurance, the researchers designed a new information acquisition system based on compressed sensing theory.
The motion data collected by the device needs to be "compressed and sampled" before sending. This ensures that the amount of data transmitted through the ZigBee wireless protocol is greatly reduced. On the computer side, there is a "signal reconstruction" link, which uses compressed sensing theory to restore the compressed data to the original signal. By avoiding high-speed sampling, the system on the one hand significantly reduces the data storage and transmission costs, on the other hand it also provides a new way for high-dimensional data analysis.
The sparsity analysis results show that the stroke signal recorded by the wearable device during sparse training has sparseness, so it can be compressed using compressed sensing. The experimental results show that the direct feature extraction in the compressed domain, and the subsequent signal processing, can avoid the signal reconstruction in the traditional framework of compressed sensing theory. While reducing the reconstruction process calculation, it also makes the next step It is possible to implement "On Node Analysis" on wearable sensor hardware. The research was published in the Sensors magazine (Volume 16, Issue 2).
(Original title: Suzhou Medical Laboratory developed wearable MEMS sensor device)